Chronic Condition Data Warehouse User Manual
3 Chronic Condition Data Warehouse n As a byproduct of administering the Medicare program, CMS has a wealth of data on program enrollment, health care utilization, and cost n Historically CMS data has been in “stovepipes”and can be very difficult to use. FFS Medicare 30 Day Readmission Rate PUF. The primary data source for these data is the CMS Chronic Condition Data Warehouse (CCW), a database with 100% of Medicare enrollment and fee-for-service claims data. In a more consumable and user friendly format. You can also edit metadata from this page. Take me there! Created Jan 17 2017. The following user guides provide information that may be helpful in understanding and analyzing the CCW data including descriptions of the CCW population, chronic condition definitions, and limitations of the data. CCW Medicare Administrative Data User Guide; CCW Medicare Encounter Data User Guide; CCW Medicare Part D Data User Guide.
- Chronic Conditions Data Warehouse » Condition Categories The CCW includes variables for 66 conditions – 27 common chronic conditions and 40 other chronic or potentially disabling conditions, which identify additional chronic health, mental health and substance abuse conditions.
- MMLEADS v2.0 User Guide (June, 2017) (PDF) MMLEADS Data Dictionaries More information on how to request MMLEADS. Chronic Condition Data Warehouse (CCW) Condition Flags (CCW Condition Flags) CMS' database of multiple chronic condition indicators or variables (i.e., 'flags') on all beneficiaries in the Medicare, Medicaid and Medicare-Medicaid.
Abstract
Many clinical practice guidelines (CPGs) are intended to provide evidence-based guidance to clinicians on a single disease, and are frequently considered inadequate when caring for patients with multiple chronic conditions (MCC), or two or more chronic conditions. It is unclear to what degree disease-specific CPGs provide guidance about MCC. In this study, we develop a method for extracting knowledge from single-disease chronic condition CPGs to determine how frequently they mention commonly co-occurring chronic diseases. We focus on 15 highly prevalent chronic conditions. We use publicly available resources, including a repository of guideline summaries from the National Guideline Clearinghouse to build a text corpus, a data dictionary of ICD-9 codes from the Medicare Chronic Conditions Data Warehouse (CCW) to construct an initial list of disease terms, and disease synonyms from the National Center for Biomedical Ontology to enhance the list of disease terms. First, for each disease guideline, we determined the frequency of comorbid condition mentions (a disease-comorbidity pair) by exactly matching disease synonyms in the text corpus. Then, we developed an annotated reference standard using a sample subset of guidelines. We used this reference standard to evaluate our approach. Then, we compared the co-prevalence of common pairs of chronic conditions from Medicare CCW data to the frequency of disease-comorbidity pairs in CPGs.
Our results show that some disease-comorbidity pairs occur more frequently in CPGs than others. Sixty-one (29.0%) of 210 possible disease-comorbidity pairs occurred zero times; for example, no guideline on chronic kidney disease mentioned depression, while heart failure guidelines mentioned ischemic heart disease the most frequently. Our method adequately identifies comorbid chronic conditions in CPG recommendations with precision 0.82, recall 0.75, and F-measure 0.78. Our work identifies knowledge currently embedded in the free text of clinical practice guideline recommendations and provides an initial view of the extent to which CPGs mention common comorbid conditions. Knowledge extracted from CPG text in this way may be useful to inform gaps in guideline recommendations regarding MCC and therefore identify potential opportunities for guideline improvement.
Introduction
Multiple chronic conditions (MCC) commonly refers to the existence of two or more chronic conditions in the same patient. More than two-thirds of Medicare beneficiaries have MCC in 2010,1 and among Veterans, more than one-third of Veterans 65 years and above have three or more chronic conditions. Providing guideline-concordant, patient-centered care to patients with MCC is challenging because many clinical practice guidelines (CPGs) typically focus on a single disease. Applying single-disease guidelines to patients with MCC increases medication treatment and self-management complexity, risk of interactions between guideline recommendations, and potential adverse events, hospitalization, and poorer outcomes.–
Suggested CPG improvements towards meaningful guidance in managing patients with MCC include cross-referencing single-disease guidelines and developing a clearer understanding of common chronic condition clusters.– However, the extent to which CPGs mention comorbid chronic conditions is unclear; and, when this has been done, such investigation has been done manually and for a single condition, such as diabetes. Reviewing all guideline recommendations for multiple chronic conditions is potentially labor-intensive, and we sought to automate the process of identifying chronic conditions in CPG text. In addition to cross-referencing comorbid chronic disease guidelines, it has also been suggested that prevalence of MCC may be able to guide the development of the next generation of CPGs in explicitly addressing common comorbid condition clusters. The frequency with which guidelines explicitly mention comorbid chronic conditions and its relationship to the prevalence of MCC has not been measured.
In this paper, we developed an automated method to assess how frequently guidelines mention highly prevalent, co-occurring chronic diseases. We performed textual annotation of CPG recommendations using clinical ontologies. Ontologies, which are machine-understandable descriptions of objects in a domain, have been widely used to codify knowledge, annotate textual documents, and perform statistical analyses. Additionally, annotation of clinical notes and electronic health record documents, published scientific literature, clinical trials announcements, and drug labels using ontologies is well-established.– We focused on 15 common chronic conditions: hypertension, diabetes mellitus, hyperlipidemia, stroke, asthma, atrial fibrillation, Alzheimer’s dementia and senile dementias, osteoporosis, chronic obstructive pulmonary disease, depression, chronic kidney disease, heart failure, arthritis, ischemic heart disease, and obesity. To our knowledge, there are no studies that automate the identification of comorbid diseases in the text of disease-specific guidelines. This approach may offer a novel opportunity to understand and extract knowledge from the text of guideline recommendations. Making such clinical practice guideline knowledge more readily accessible may support efforts towards retrieving guideline knowledge for use by clinicians in decision-making, developers of clinical decision support systems in personalizing care for patients with MCC, and institutional guideline implementation working groups and committees in managing knowledge. We compared the frequency of disease-comorbidity pairs to the prevalence of these comorbid condition pairs in the Medicare population.
Materials and Methods
To analyze CPG text, we used three publicly available repositories of information: (1) guideline summaries from the National Guideline Clearinghouse, (2) epidemiologic data on the co-prevalence of chronic diseases and a data dictionary of chronic conditions from the Medicare Chronic Conditions Data Warehouse, and (3) biomedical ontologies in the Bioportal repository of the National Center for Biomedical Ontology.
Data Sources
National Guideline Clearinghouse
The Agency for Healthcare Research and Quality maintains the National Guideline Clearinghouse (NGC), first developed in 1997, which identifies published CPGs according to specified inclusion criteria, creates guideline summaries that contain structured, standardized sections, and archives them in a publicly accessible website for retrieval.22 NGC guideline summaries are structured using 54 guideline attributes,23 such as Guideline Title, Guideline Objective, and Major Recommendations.23 As of the end of February 2014, the NGC featured more than 2,500 guidelines.
National Center for Biomedical Ontology
The National Center for Biomedical Ontology (NCBO) is an international consortium based at Stanford University, funded by the National Institutes of Health, which provides online tools for accessing and integrating ontological resources, including Bioportal, a repository of biomedical ontologies. As of February 2014, the NCBO Bioportal contained nearly 400 biomedical ontologies. For this study, we utilized Bioportal ontologies to identify disease synonyms for the 15 chronic conditions of interest.
Medicare Chronic Conditions Data Warehouse
The Chronic Conditions Data Warehouse (CCW), administered by the Centers for Medicare and Medicaid Services, is a research database of care provided to Medicare beneficiaries with chronic conditions. The CCW contains a data dictionary that we used to create a list of ICD-9 (9th version of the International Statistical Classification of Diseases and Related Health Problems) codes for 14 of the most prevalent chronic diseases among Medicare beneficiaries: hypertension, diabetes mellitus, hyperlipidemia, stroke, asthma, atrial fibrillation, Alzheimer’s dementia and senile dementias, osteoporosis, chronic obstructive pulmonary disease, depression, chronic kidney disease, heart failure, arthritis, and ischemic heart disease.24 We added 3 more ICD-9 codes for obesity, which we added as the 15th disease due to its high prevalence and clinical significance, instead of cancer, which is more heterogeneous. The CCW also provided publicly accessible data on the co-prevalence of chronic conditions in the Medicare population.
Guideline Recommendations Corpus
We compiled a corpus of text from the NGC by downloading the XML version of 2,503 unique guideline summaries in March 2014. We obtained the Major Recommendation sections from 268 XML-based NGC guideline summaries. CPGs were included if (1) at least one of the 15 diseases was mentioned in the title and (2) the target population was the general adult, non-pregnant population. We excluded guideline summaries that were not relevant to the 15 chronic conditions of interest based on the guideline title. For those guidelines that were subdivided into separate summaries, we combined these into a single summary; for example, the American Diabetes Association publishes a full guideline document entitled the Standards of Medical Care in Diabetes, which the NGC parses into nine separate guideline summaries.22 In this study we refer to NGC guideline summaries as clinical practice guidelines, or CPGs.
Algorithm
We first constructed a list of 448 ICD-9 codes for the 15 diseases using the Medicare CCW.24 In addition, we identified three corresponding ICD-9 codes for obesity (Figure 1). Then, we used the NCBO Bioportal Representational State Transfer (REST) services to obtain 1,829 unique preferred labels and synonyms for each ICD-9 code. Finally, we developed a text-mining algorithm to identify disease mentions by exactly matching the preferred labels and synonyms in the text corpus. All algorithms were developed using Python 2.7.8.
Annotation pipeline for automated identification of disease labels for 15 chronic conditions. Labels include NCBO preferred labels and synonyms.
We counted the frequency of chronic condition mentions in the CPG recommendations (a disease-comorbidity pair). Specifically, the topic for each disease guideline was the disease in the disease-comorbidity pair, and a mention of a comorbid chronic condition was the comorbidity. Each guideline might have more than one instance of a disease-comorbidity pair if the recommendation text mentioned more than one exact match of the comorbid disease term. For example, in a heart failure guideline, if coronary artery disease and acute myocardial infarction are each mentioned one time, then there would be two occurrences of the heart failure-ischemic heart disease pair in this guideline.
Now, you could purchase a Clymer service manual from Amazon, or an OEM factory service manual which will cost way more. In the video below, I’ll walk you through the process of locating a FREE service manual download. Disclaimer: I do not download any free service manuals. I prefer to purchase a. Yes, the online/digital format does provide a number of advantages. All the same great content as the print manual, only better! You can use the online manual on many devices such as a laptop, tablet or even a smartphone. Photos, illustrations and diagrams are in color. The vehicle service manuals you’ve come to know and trust are now available for online viewing. Your online repair manual is viewable with a magazine reader and features the same content as the printed manual. Clymer online manuals are excellent for viewing on tablets such as the iPad. The perfect tool can now be used in a mobile environment. Clymer service and repair manuals are written with model specific coverage for all your service, repair, and maintenance needs. The most important tool in your toolbox may be your Clymer manual. Can i download the clymer manual.
We evaluated our method by developing a reference standard that summarized the number of disease-comorbidity pairs in the recommendations text of a sample of guidelines. Then, precision, recall, and F-measure were calculated. To compare the prevalence of common chronic condition pairs with the disease-comorbidity frequencies in CPGs, we constructed two network maps. Network maps were used to better illustrate each condition’s prevalence and their co-occurrence with comorbid chronic conditions. We obtained epidemiologic data on prevalence and co-occurrence of 14 of the 15 diseases from the Medicare CDW; obesity co-occurrence was estimated from published literature.
Reference Standard
We developed a reference standard to identify comorbid disease concepts in a sample subset of CPG text (Figure 2). In the sample, we randomly selected one guideline for each chronic condition for a total of 15 guidelines manually annotated. As an annotation guide for manual annotation, comorbid disease mentions in each guideline, included (1) explicit comorbid disease mentions, including common synonyms (e.g. left ventricular systolic failure for congestive heart failure) or guideline-defined acronyms (e.g. CHF for chronic heart failure) and (2) statements that referenced or described a mention of one of the 15 chronic conditions, or for which the mention could be inferred from the clinical context of the statement. For example, one coronary artery disease guideline states: “Individuals with established cardiovascular disease, who also have chronic renal disease or diabetes with complications, or target organ damage may be considered for treatment at the lower threshold of systolic 130 mm Hg and/or diastolic 80 mm Hg.”27 This statement mentions a description clinically consistent with hypertension, even if hypertension is not explicitly stated.
Development of annotation guide and reference standard
Two manual annotators with medical expertise (TL and HJ) performed the annotation using the guide, adjudicated discrepancies, revised the guide and finalized the reference standard. We determined interannotator agreement (IAA) by calculating a ratio of the number of sentence agreements to the total number of sentences, and Cohen’s Kappa (κ).
Results
Selection of guideline summaries meeting criteria for inclusion in the text corpus are illustrated in Figure 3. To ensure we included all relevant titles, we performed both a text search and a manual review of all 2,503 unique guideline summary titles. After inclusion based on text search of the titles, manual review of the titles identified 26 additional guideline summaries where it was unclear if it met inclusion criteria; we manually reviewed the Major Recommendations to identify the disease, and included 23 guideline summaries.
Guideline summaries meeting inclusion criteria for text corpus. CPG = clinical practice guideline
In the reference standard, manual annotators agreed on annotations for 2,890 (97.0%) of 2,981 sentences. In this preliminary evaluation, compared to the reference standard, precision of our approach was 0.82, recall was 0.75, and F-measure was 0.78.
Figure 4 summarizes our preliminary findings on the number of CPGs for each condition (represented by each node) and the number of disease-comorbidity pairs (represented by a directed edge). For example, the diabetes mellitus-ischemic heart disease pair occurs 153 times in 43 diabetes CPGs; conversely, the ischemic-heart disease-diabetes mellitus pair occurs 323 times in 38 ischemic heart disease CPGs. CPGs for concordant diseases (diseases that are part of the same pathophysiologic risk profile), such as hypertension, diabetes, and ischemic heart disease, mentioned one another most frequently. Hypertension was the only disease mentioned across all CPGs, while Alzheimer’s disease and osteoporosis were mentioned the least. Figure 5 illustrates the prevalence of each chronic disease among Medicare beneficiaries in 2012 (nodes), and the co-occurrence of each condition with another common chronic condition (edges). For example, the prevalence of diabetes mellitus and Alzheimer’s disease and dementia are 18% and 11%, respectively, and the prevalence of their co-occurrence is 5.4%.
Frequency of disease-comorbidity pairs
Co-occurring chronic diseases among Medicare beneficiaries, 2012
The network diagrams of disease-comorbidity pairs and the prevalence of co-occurring chronic condition pairs highlights a mismatch between explicit mentions of chronic conditions in CPGs and the prevalence of MCC in Medicare beneficiaries. The mismatch is less pronounced for concordant chronic conditions, such as hypertension, diabetes, and hyperlipidemia. For discordant conditions, the mismatch is more pronounced, such as in hypertension and arthritis, which co-occur in the Medicare population with a prevalence of 32.2%, but have minimal explicit mentions of one another: the arthritis-hypertension pair occurs 5 times in 19 arthritis CPGs and hypertension-arthritis pair occurs zero times in 13 hypertension CPGs.
In general, some disease-comorbidity pairs occur more frequently in CPGs than others. Sixty-one (29.0%) of 210 possible disease-comorbidity pairs occurred zero times; for example, no guideline on chronic kidney disease mentioned depression, while heart failure guidelines mentioned ischemic heart disease 209 times, the most frequently occurring disease-comorbidity pair among included CPGs.
Discussion
We developed an automated method that identifies comorbid conditions in CPG recommendations. Our results suggest that highly prevalent co-occurring pairs of conditions are variably addressed explicitly in corresponding disease guidelines. For concordant conditions, which also co-occur commonly in the Medicare population, the high frequency of disease-comorbidity pairs suggest that more guidance exists in CPG recommendations for these combinations of MCC. However, for discordant conditions, less guidance may exist, although such conditions commonly co-occur among patients with MCC. These represent potentially high-impact areas of opportunity for guideline improvement in order to provide clinicians with explicit guidance on providing care for these patients with common MCC combinations.
Chronic Conditions Data Warehouse 2018
Limitations of this study include the use of NGC guideline summaries, rather than original, full-text CPGs. Additionally, the text corpus is small, totaling only 268 guideline summaries. In a larger text corpus inclusive of full-text guidelines, disease-comorbidity pairs may occur more frequently and potentially provide a more accurate representation of MCC in CPG recommendations. A more extensive evaluation of our method using a reference standard including more CPGs also could be informative, allowing for a more detailed understanding of how the method performs for the identification of each chronic condition in CPG text. Future research could iteratively improve the text-mining algorithm developed, explore alternative analytic methods, evaluate alternative annotation tools for biomedical concept annotation, and perform natural language processing on the text corpus.
Further investigation is needed to understand the observed variation in the frequency of disease-comorbidity pairs chronic disease CPGs. Our methods can be used to extract knowledge from CPGs about chronic conditions towards facilitating future research and identify guideline improvement opportunities. Making such clinical practice guideline knowledge more readily accessible may support efforts towards retrieving guideline knowledge for use by clinicians in managing care for patients with MCC, developing clinical decision support systems personalizing care for patients with MCC, and assisting institutional guideline working groups in managing knowledge.
Conclusion
Our approach adequately identifies explicit knowledge about comorbid chronic conditions currently embedded in the free text of clinical practice guideline recommendations. Our findings may help prioritize opportunities for single-disease chronic condition guidelines to be improved towards providing specific guidance for patients with MCC, especially for discordant comorbid conditions.
Acknowledgments
Drs. Leung and Jalal were supported by the Veterans Affairs Advanced Fellowship in Medical Informatics. Views expressed are those of the authors and not necessarily those of the Department of Veterans Affairs.
The authors thank Ray Fergerson, PhD, Project Director, National Center for Biomedical Ontology; Mary Nix, MS, Lisa Haskell, MS, National Guideline Clearinghouse, AHRQ; Vivian Coates, MBA, Eileen Erinoff, BA, ECRI Institute
References
Chronic Conditions Warehouse Cms
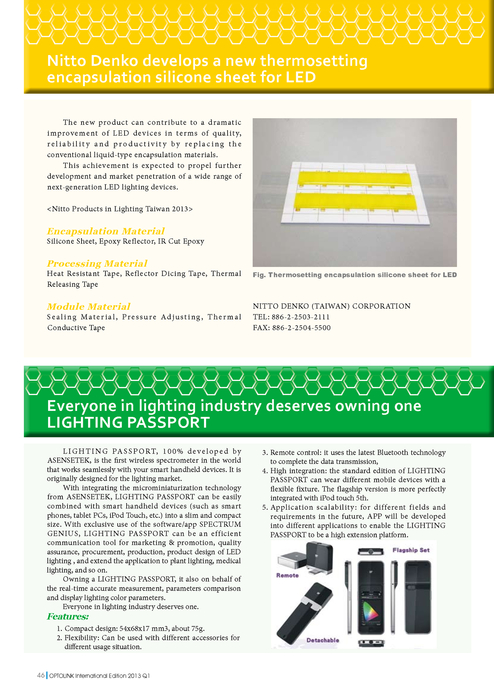